Distinguished Lectures
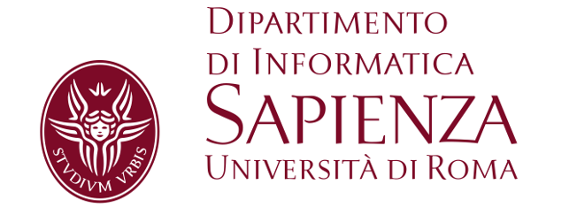
The Computer Science Department at Sapienza University of Rome is promoting a series of Distinguished Lectures held by renowned speakers on fundamental research topics in computer science. The goal of each lecture (approx. 45 minutes) is to explain why the theme is indeed fundamental, and to summarize the state of the art up to cutting edge research.
Supported by MIUR under grant "Dipartimenti di eccellenza 2018-2022", of the Computer Science Department at Sapienza University.
2024
Lecturer: Prof. Alexandra Silva
Title: The Frontiers of Active Learning in Network Verification.
Location: Aula 101 (Palazzina D) - Viale Regina Elena 295
Date and time:
Monday, November 25, 12:00-13:00
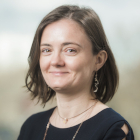
Lecturer: Prof. Michael Swift
Title: Virtual Memory in the 21st century
Location: Aula 101 (Palazzina D) - Viale Regina Elena 295
Date and time:
Wednesday, May 08, 12:00-13:00
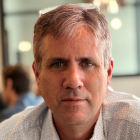
Lecturer: Prof. Monika Henzinger
Title: How Can Algorithms Help in Protecting our Privacy
Location: Aula 201 - Viale Regina Elena 295
Date and time:
Tuesday March 12, 12:00-13:00
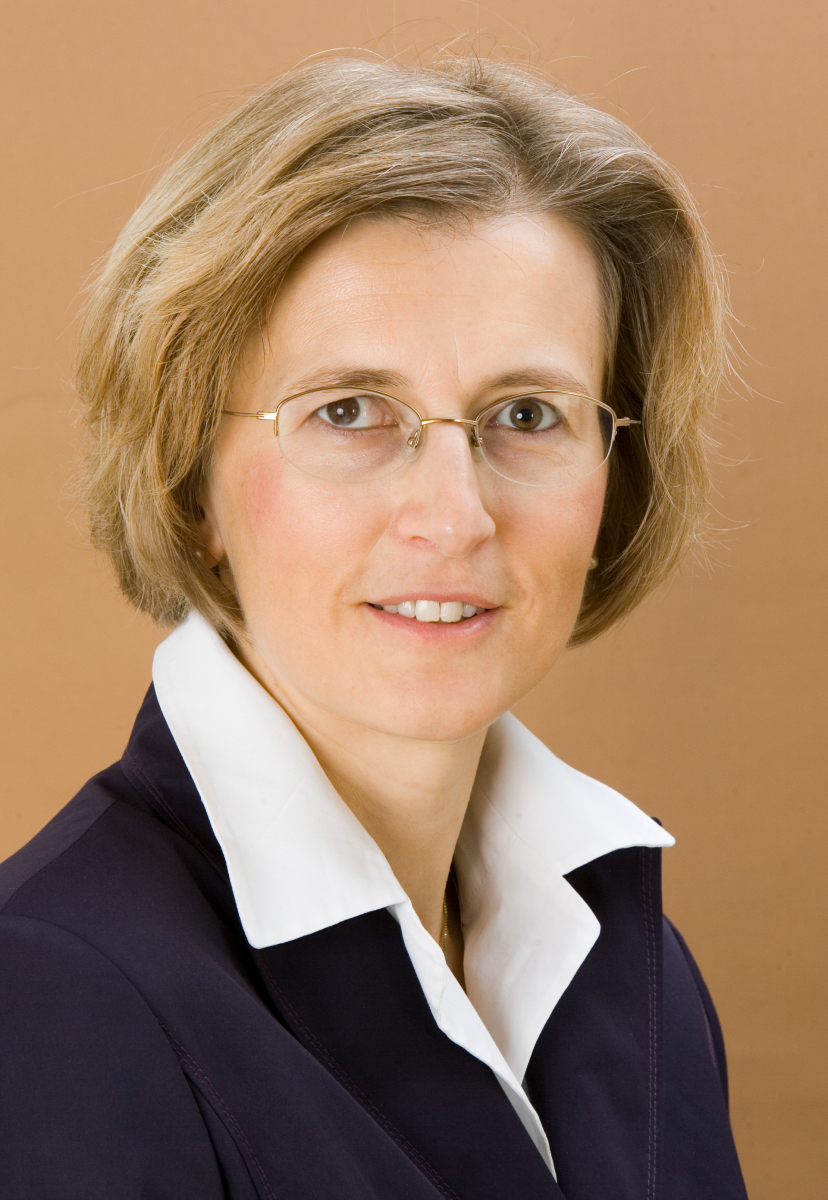
- 2023
-
2023
Lecturer: Prof. Ronitt Rubinfeld
Title: Making use of locality in computation
Location: Aula 101, Palazzina D, Viale Regina Elena 295
Date and time:
Monday October 23, 12:00-13:00Ronitt Rubinfeld is the Edwin Sibley Webster Professor in MIT's Electrical Engineering and Computer Science department. Her research centers on property testing and sub-linear time algorithms, that provide the foundations for measuring the performance of algorithms that analyze data by looking at only a very small portion of it. She was an ONR Young Investigator, a Sloan Fellow, and an invited speaker at the International Congress of Mathematicians in 2006. She is a fellow of the ACM, a fellow of the American Academy of Arts and Sciences, and a member of the National Academy of Sciences. She received the STOC 30 year test of time award for her work with Blum and Luby on "Self-testing/correcting with applications to numerical problems".Abstract. Modern networks can be such that complete inputs and outputs for computational problems on them are *so large*, that there is not time to read or write them in their entirety. However, if one is only interested in small parts of the output at any given time, is it really necessary to solve the entire computational problem? Can one avoid the need to view the whole input? Or, is there a way to take advantage of certain "locality properties" of the problem?
We describe recent work in the model of "local computation algorithms" which for a given input, supports queries by a user to values of specified bits of a legal output. The goal is to design local computation algorithms in such a way that very little of the input needs to be seen in order to determine the value of any single bit of the output. Though this model describes sequential computations, techniques from local distributed algorithms have been extremely important in designing efficient local computation algorithms. We describe results on a variety of problems for which sublinear time and space local computation algorithms have been developed.Lecturer: Prof. Boaz Barak
Title: The uneasy relation between deep learning and statistics
Location: Aula 201, Palazzina D, Viale Regina Elena 295
Meet zoomDate and time:
Tuesday June 20, 12:00-13:00
Boaz Barak is the Gordon McKay professor of Computer Science at Harvard University's John A. Paulson School of Engineering and Applied Sciences. Barak's research interests include all areas of theoretical computer science and in particular cryptography, computational complexity, and the foundations of machine learning. Previously, he was a principal researcher at Microsoft Research New England, and before that an associate professor (with tenure) at Princeton University's computer science department. Barak has won the ACM dissertation award, the Packard and Sloan fellowships, and was also selected for Foreign Policy magazine's list of 100 leading global thinkers for 2014. He was also chosen as a Simons investigator and a Fellow of the ACM. Barak is a member of the scientific advisory boards for Quanta Magazine and the Simons Institute for the Theory of Computing. He is also a board member of AddisCoder, a non-profit organization for teaching algorithms and coding to high-school students in Ethiopia and Jamaica. Barak wrote with Sanjeev Arora the textbook "Computational Complexity: A Modern Approach".Abstract. Deep learning uses the language and tools of statistics and classical machine learning, including empirical and population losses and optimizing a hypothesis on a training set. But it uses these tools in regimes where they should not be applicable: the optimization task is non-convex, models are often large enough to overfit, and the training and deployment tasks can radically differ.
In this talk I will survey the relation between deep learning and statistics. In particular we will discuss recent works supporting the emerging intuition that deep learning is closer in some aspects to human learning than to classical statistics. Rather than estimating quantities from samples, deep neural nets develop broadly applicable representations and skills through their training.
The talk will not assume background knowledge in artificial intelligence or deep learning.Lecturer: Prof. Keshav Pingali
Title: Fifty Years of Parallel Programming: Ieri, Oggi, Domani
Location: Aula Aldo La Ginestra, Edificio di Chimica (Città Universitaria), Piazzale Aldo Moro, 5
Date and time:
Monday March 20th, 12:00-13:00
Keshav Pingali is a Professor in the Department of Computer Science at the University of Texas at Austin, where he holds the W.A."Tex" Moncrief Chair of Computing in the Institute for Computational Engineering and Sciences (ICES) at UT Austin. He was on the faculty of the Department of Computer Science at Cornell University from 1986 to 2006, where he held the India Chair of Computer Science. He has a PhD from MIT, and a B.Tech. from the Indian Institute of Technology, Kanpur, where he was awarded the President's Gold Medal.
Pingali's research has focused on programming languages and compiler technology for program understanding, restructuring, and optimization. His group is known for its contributions to memory-hierarchy optimization; some of these have been patented and are in use in industry compilers. His current research is focused on programming models and tools for high-performance graph computing.
Pingali is a Fellow of the IEEE, ACM and AAAS. He received the IIT Kanpur Distinguished Alumnus Award in 2013, and the IEEE CS Charles Babbage Award in 2023.
Between 2008 and 2011, he was the co-Editor-in-chief of the ACM Transactions on Programming Languages and Systems. He has also served on the NSF CISE Advisory Committee. He is currently the CEO of Katana Graph, a start-up in the graph computing area backed by leading investors from Silicon Valley.Abstract. Parallel programming started around 1970 so as a discipline, it is now more than 50 years old. What have we learned in the past 50 years about parallel programming? What problems have we solved and what problems remain to be solved? What can young researchers learn from the successes and failures of our discipline? This talk presents a personal point of view about these and other questions regarding the state of parallel programming. I will also discuss my experience in doing a start-up called Katana Graph, which is based on my work in parallel computing. - 2022
-
2022
Lecturer: Prof. Vitaly Shmatikov
Title: Can We Trust Machine Learning Models?
Location: Aula 201, Palazzina D, Viale Regina Elena 295
Date and time:
Wednesday 14th December, 15:00 - 16:00
Vitaly Shmatikov is a professor of computer science at Cornell Tech, where he works on computer security and privacy. Vitaly's research team has received the PET Award for Outstanding Research in Privacy Enhancing Technologies three times, as well as multiple Distinguished Paper and Test-of-Time Awards from the IEEE Symposium on Security and Privacy, USENIX Security Symposium, and the ACM Conference on Computer and Communications Security. Prior to joining Cornell, Vitaly worked at the University of Texas at Austin and SRI International.
Abstract. Modern machine learning models achieve super-human accuracy on tasks such as image classification and natural-language generation, but accuracy does not tell the entire story of what these models are learning. In this talk, I will look at today's machine learning from a security and privacy perspective, and ask several fundamental questions. Could models trained on sensitive private data memorize and leak this data? When training involves crowd-sourced data, untrusted users, or third-party code, could models learn malicious functionality, causing them to produce incorrect or biased outputs? What damage could result from such compromised models?I will illustrate these vulnerabilities with concrete examples and discuss the benefits and tradeoffs of technologies (such as federated learning) that promise to protect the integrity and privacy of machine learning models and their training data. I will then outline practical approaches towards making trusted machine learning a reality.
Lecturer: Prof. Avi Wigderson
Title: Imitation Games
Location: Zoom Meeting
Date and time:
Wednesday, October 28th, 16:30 - 17:30
Meeting recording (passcode: &.!B31Fa)Avi Wigderson is the Herbert H. Maass Professor in the School of Mathematics at the Institute for Advanced Study. Wigderson earned his Ph.D. from Princeton University in 1983.His research covers many aspects of computational complexity theory and its interactions with mathematics, including randomness and pseudorandomness; algorithms and optimization; circuit and proof complexity; quantum computation and communication; and cryptography.
Professor Wigderson has received many awards for his work in the field including the 2021 Abel Prize, Knuth Prize, Gödel Prize, Conant Prize, and Nevanlinna Prize. He is also a member of the American Academy of Arts and Sciences, National Academy of Science and the Norwegian Academy of Science and Letters.
Abstract. One of Alan Turing's most influential papers is his 1950 Computing machinery and intelligence, in which he introduces the famous "Turing test" for probing the nature of intelligence by evaluating the abilities of machines to behave as humans. In this test, which he calls the "Imitation Game," a (human) referee has to distinguish between two (remote and separate) entities, a human and a computer, only by observing answers to a sequence of arbitrary questions to each entity.This lecture will exposit, through examples from a surprisingly diverse array of settings, the remarkable power of this basic idea to understand many other concepts. I will discuss variations of the Imitation Game in which we change the nature of the referee, and of the objects to be distinguished, to yield different analogs of the Turing test. These new Imitation Games lead to novel, precise, and operative definitions of classical notions, including secret, knowledge, privacy, randomness, proof, fairness, and others. These definitions have in turn led to numerous results, applications, and understanding. Some, among many consequences of this fundamental paradigm, are the foundations of cryptography, the surprising discoveries on the power and limits of randomness, the recent influential notion of differential privacy, and breakthrough results on patterns in the prime numbers and navigation in networks. Central to each of these settings are computational and information theoretic limitations placed on the referee in the relevant Imitation Game.
This lecture will survey some of these developments and speculate on future uses of this paradigm in science and society, in a way which is hopefully accessible without any specific background knowledge.
Lecturer: Prof. Hajo A. Reijers
Title: Process Science: Past, Present, Future
Location: Aula Alfa, Via Salaria 113
Date and time:
Tuesday, May 17th, 10:00 – 12:00
Wednesday, May 18th, 16:30 – 17:30Hajo Reijers is a full professor in the Department of Information and Computing Sciences of Utrecht University, where he leads the Business Process Management & Analytics group. He is also a part-time, full professor in the Department of Mathematics and Computer Science of Eindhoven University of Technology (TU/e) and an adjunct professor in the School of Information Systems, Science and Engineering Faculty, at Queensland University of Technology.Previously, Hajo worked in industry as a consultant for Bakkenist Management Consultants and Accenture, where he was involved in various reengineering projects and workflow system implementations. Hajo led the BPM research group at Lexmark from 2012 to 2014. From 2014 to 2018, he was appointed as a full professor in the Department of Computer Sciences at the Vrije Universiteit Amsterdam. He has been an advisor to various start-ups in the high-tech sector.
The focus of Hajo's academic research is on business process redesign, process automation, conceptual modeling, and enterprise information systems. On these topics, he contributed to about 300 scientific papers, chapters in edited books, and articles in professional journals.
During the spring of 2022, Hajo is a visiting professor at the Department of Computer Science of the Sapienza University of Rome, where he can be reached through reijers@di.uniroma1.it .
Abstract. In 2021, a proposal was launched to establish "process science", a multi-disciplinary field for the study of processes [1]. Processes are coherent series of changes, both man-made and naturally occurring, which unfold over time and occur at various levels. Process science is concerned with discovering and understanding processes as well as designing interventions to shape them into desired directions. Computer science, engineering, and data science greatly contribute to this field, but there are also strong relations with economics, management science, organization science, and the social sciences.In this PhD seminar, I will give a personal reflection on the past, the present, and the future of the discipline. In the part of the seminar that is scheduled for May 17th, I will first sketch the historic development of process science and next provide a summary of its major accomplishments. In the follow-up talk on May 18th, I will outline some of the major challenges that we face, which hopefully can serve as in inspiration for researchers at all levels and from a variety of backgrounds to contribute to this emerging and exciting field.
[1] vom Brocke, J., van der Aalst, W.M.P, Grisold, T., Kremser, W., Mendling, J., Pentland, B., Recker, J., Roeglinger, M., Rosemann, M. Weber, B. (2021). Process Science: The Interdisciplinary Study of Continuous Change. Working Paper, available at SSRN Electronic Library, 2021.
- 2021
-
2021
Lecturer: Lorenzo Alvisi
Title: Order! A tale of money, intrigue, and specifications
Location: Aula Alfa, Via Salaria 113
Zoom meeting
Date: December 2, 2021.
Time: 16.00-17.00 (CET)Lorenzo Alvisi is the Tisch University Professor of Computer Science at Cornell University. Prior to joining Cornell, he held an endowed professorship at UT Austin, where he is now a Distinguished Professor Emeritus. Lorenzo received his Ph.D. in 1996 from Cornell, after earning a Laurea cum Laude in Physics from the University of Bologna. His research interests are in the theory and practice of distributed computing. He is a Fellow of the ACM and IEEE, an Alfred P. Sloan Foundation Fellow, and the recipient of a Humboldt Research Award, an NSF Career Award, and several teaching awards. He serves on the editorial boards of ACM TOCS and Springer's Distributed Computing. In addition to distributed computing, he is passionate about classical music and red Italian motorcycles.
Abstract. Mistrust over traditional financial institutions is motivating the development of decentralized financial infrastructures based on blockchains. In particular, Consortium blockchains (such as the Linux Foundation Hyperledger and Facebook’s diem) are emerging as the approach preferred by businesses. These systems allow only a well-known set of mutually distrustful parties to add blocks to the blockchain; in this way, they aim to retain the benefits of decentralization without embracing the cyberpunk philosophy that informed Nakamoto’s disruptive vision.At the core of consortium blockchains is State Machine Replication, a classic technique borrowed from fault tolerant distributed computing; to ensure the robustness of their infrastructure, consortium blockchains actually borrow the Byzantine-tolerant version of this technique, which guarantees that the blockchain will operate correctly even if as many as about a third of the contributing parties are bent on cheating. But, sometimes, "a borrowing is a sorrowing".
I will discuss why Byzantine-tolerant state machine replication is fundamentally incapable of recognizing, never mind preventing, an ever present scourge of financial exchanges: the fraudulent manipulation of the order in which transactions are processed - and how its specification needs to be expanded to give it a fighting chance.
But is it possible to completely eliminate the ability of Byzantine parties to engage in order manipulation? What meaningful ordering guarantees can be enforced? And at what cost?
Lecturer: Jeffrey David Ullman
Title: Abstractions and Their Compilers
Location: Zoom meeting
Date: September 16, 2021.
Time: 17.00-18.00 (CET)Jeffrey David Ullman is the Stanford W. Ascherman Professor of Engineering (Emeritus) in the Department of Computer Science at Stanford and CEO of Gradiance Corp. He received the B.S. degree from Columbia University in 1963 and the PhD from Princeton in 1966. Prior to his appointment at Stanford in 1979, he was a member of the technical staff of Bell Laboratories from 1966-1969, and on the faculty of Princeton University between 1969 and 1979. From 1990-1994, he was chair of the Stanford Computer Science Department. Ullman was elected to the National Academy of Engineering in 1989, the American Academy of Arts and Sciences in 2012, the National Academy of Sciences in 2020, and has held Guggenheim and Einstein Fellowships. He has received the Sigmod Contributions Award (1996), the ACM Karl V. Karlstrom Outstanding Educator Award (1998), the Knuth Prize (2000), the Sigmod E. F. Codd Innovations award (2006), the IEEE von Neumann medal (2010), the NEC C&C Foundation Prize (2017), and the ACM A.M. Turing Award (2020). He is the author of 16 books, including books on database systems, data mining, compilers, automata theory, and algorithms.
Abstract. An abstraction in computer science is a data model plus a "programming language"; the language is often far simpler than a general-purpose programming language. We shall consider four different ways that abstractions have been used. Especially important are "declarative abstractions," where you say what you want done but not how to do it. These abstractions require clever compilation, including some powerful optimization techniques, if they are to be used in practice. We shall talk about three such declarative abstractions: regular expressions, and their compilation into finite automata, context-free grammars and their compilation into shift-reduce parsers, and the relational model of data, and its compilation into executable code.Lecturer: Michael Bronstein
Title: Geometric Deep Learning: from Euclid to drug design
Location: Zoom meeting
Date: April 27, 2021.
Time: 11.00-12.00 (CET)
Meeting recordingMichael Bronstein is a professor at Imperial College London, where he holds the Chair in Machine Learning and Pattern Recognition, and Head of Graph Learning Research at Twitter. He also heads ML research in Project CETI, a TED Audacious Prize-winning collaboration aimed at understanding the communication of sperm whales. Michael received his PhD from the Technion in 2007. He has held visiting appointments at Stanford, MIT, and Harvard, and has also been affiliated with three Institutes for Advanced Study (at TUM as a Rudolf Diesel Fellow (2017-2019), at Harvard as a Radcliffe fellow (2017-2018), and at Princeton as a short-term scholar (2020)).
Michael is the recipient of the Royal Society Wolfson Research Merit Award, Royal Academy of Engineering Silver Medal, five ERC grants, two Google Faculty Research Awards, and two Amazon AWS ML Research Awards. He is a Member of the Academia Europaea, Fellow of IEEE, IAPR, BCS, and ELLIS, ACM Distinguished Speaker, and World Economic Forum Young Scientist. In addition to his academic career, Michael is a serial entrepreneur and founder of multiple startup companies, including Novafora, Invision (acquired by Intel in 2012), Videocites, and Fabula AI (acquired by Twitter in 2019). He has previously served as Principal Engineer at Intel Perceptual Computing and was one of the key developers of the Intel RealSense technology.
Abstract. For nearly two millennia, the word "geometry" was synonymous with Euclidean geometry, as no other types of geometry existed. Euclid's monopoly came to an end in the 19th century, where multiple examples of non-Euclidean geometries were shown. However, these studies quickly diverged into disparate fields, with mathematicians debating the relations between different geometries and what defines one. A way out of this pickle was shown by Felix Klein in his Erlangen Programme, which proposed approaching geometry as the study of invariants or symmetries using the language of group theory. In the 20th century, these ideas have been fundamental in developing modern physics, culminating in the Standard Model.The current state of deep learning somewhat resembles the situation in the field of geometry in the 19h century: On the one hand, in the past decade, deep learning has brought a revolution in data science and made possible many tasks previously thought to be beyond reach -- including computer vision, playing Go, or protein folding. At the same time, we have a zoo of neural network architectures for various kinds of data, but few unifying principles. As in times past, it is difficult to understand the relations between different methods, inevitably resulting in the reinvention and re-branding of the same concepts.
Geometric Deep Learning aims to bring geometric unification to deep learning in the spirit of the Erlangen Programme. Such an endeavour serves a dual purpose: it provides a common mathematical framework to study the most successful neural network architectures, such as CNNs, RNNs, GNNs, and Transformers, and gives a constructive procedure to incorporate prior knowledge into neural networks and build future architectures in a principled way. In this talk, I will overview the mathematical principles underlying Geometric Deep Learning on grids, graphs, and manifolds, and show some of the exciting and groundbreaking applications of these methods in the domains of computer vision, social science, biology, and drug design.
(based on joint work with J. Bruna, T. Cohen, P. Veličković)
Lecturer: Alessandro Chiesa
Title: From Zero Knowledge to Private Transactions
Location: Zoom meeting
Date: February 24, 2021.
Time: 17.00-18.00 (CET)
SlidesAlessandro Chiesa is a faculty member in computer science at UC Berkeley. He conducts research in complexity theory, cryptography, and security, with a focus on the theoretical foundations and practical implementations of cryptographic proofs that are short and easy to verify. He is a co-author of several zkSNARK libraries, and is a co-inventor of the Zerocash protocol. He has co-founded Zcash and StarkWare Industries. He received S.B. degrees in Computer Science and in Mathematics, and a Ph.D. in Computer Science, from MIT.
Abstract. The integrity of digital currencies such as Bitcoin rests on the fact that every payment is broadcast in plaintext. This fails to provide any meaningful privacy guarantees for users. In this talk I will explain how a beautiful cryptographic tool, zero knowledge proofs, can be used to design digital currencies that provide strong privacy guarantees for users. These ideas have been deployed in the wild, as part of the cryptocurrency Zcash and in several other settings. - 2020
-
2020
Lecturer: Luciano Floridi
Title: AI, Digital Utopia, and “Asymptopia”
Location: Zoom meeting
Date: December 1, 2020.
Time: 11.00-12.00 (CET)Luciano Floridi Professor of Philosophy and Ethics of Information at the University of Oxford, where he is Director of the OII Digital Ethics Lab. He is a world-renowned expert on digital ethics, the ethics of AI, the philosophy of information, and the philosophy of technology. He has published more than 300 works, translated into many languages. He is deeply engaged with policy initiatives on the socio-ethical value and implications of digital technologies and their applications, and collaborates closely on these topics with many governments and companies worldwide.
Abstract. AI promises to be one of the most transformative technologies of our age. In this lecture, I will argue that AI can actually support the development of a better future, both for humanity and for the planet. In the course of the lecture, I will outline the nature of utopian thinking, the relationship between it and digital technologies, and introduce what I shall call “asymptopia”, or asymptotic utopia, the possibility of a progressive improvement of our society steered by regulative ideals (Kant).Lecturer: Luca Trevisan
Title: P versus NP
Location: Aula Seminari, Via Salaria 113, 3rd Floor.
Date: February 6, 2020.
Time: 11.30-12.30Luca Trevisan is a professor of Computer Science at Bocconi University. Luca studied at the Sapienza University of Rome, he was a post-doc at MIT and at DIMACS, and he was on the faculty of Columbia University, U.C. Berkeley, and Stanford, before returning to Berkeley in 2014 and, at long last, moving back to Italy in 2019.Luca's research is focused on computational complexity, on analysis of algorithms, and on problems at the intersection of pure mathematics and theoretical computer science.
Luca received the ACM STOC'97 Danny Lewin (best student paper) award, the 2000 Oberwolfach Prize, and the 2000 Sloan Fellowship. He was an invited speaker at the 2006 International Congress of Mathematicians. He is a recipient of a 2019 ERC Advanced Grant.
Abstract. The P versus NP problem asks whether every time we have an efficient algorithm to verify the validity of a solution for a computational problem we must also have an efficient algorithm to construct such a valid solution. It is one of the major open problems in mathematics and computer science and one of the six unresolved "Millenium Problems" with a million dollar prize on its solution.We will trace the origin of this question, and its conceptual implications about the nature of mathematical proofs and the notions of expertise and creativity. We will see why certain proof strategies cannot possibly resolve this problem, and why fundamentally different ideas are needed to make progress. Finally, we will discuss "average-case analysis" extensions of the P versus NP problems, which are needed to reason about the security of cryptographic protocols and blockchains and about the complexity of problems arising in machine learning.